When Graph Neural Networks Meet Reinforcement Learning
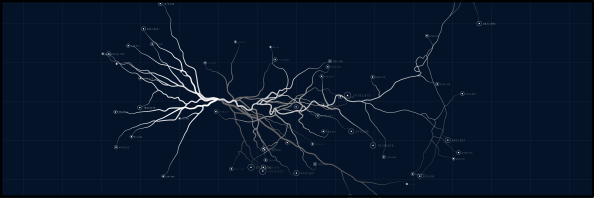
Reinforcement Learning (RL) agents should be able to efficiently generalize to novel situations and transfer their learned skills. Without these properties, such agents would always have to learn from scratch, even though they have already mastered primitive skills that could potentially be leveraged to acquire more complex ones.